Application Areas
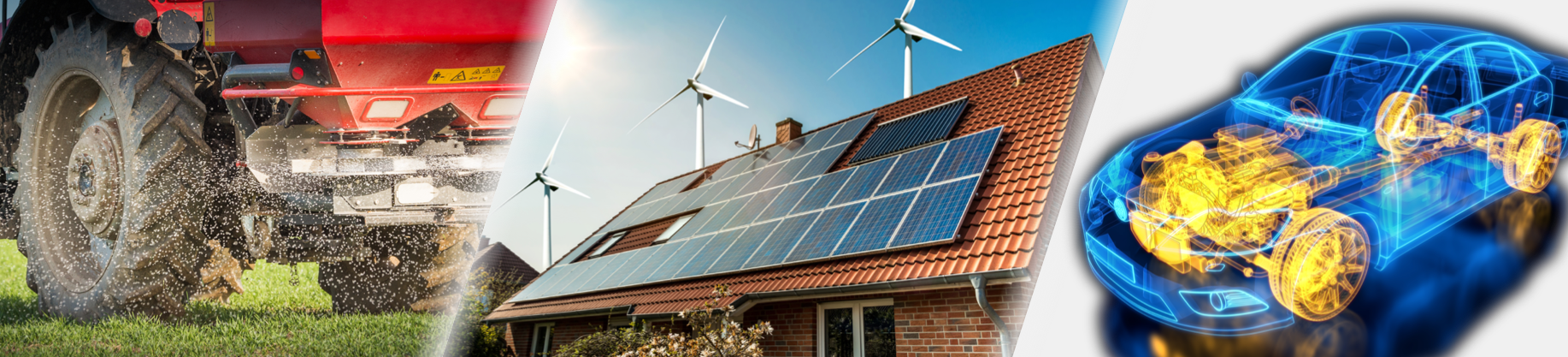
The core research methods of the lab are applied mainly in the following areas:
- Agricultural Systems
- Energy Systems
- Vehicle Control
Selected projects

The energy revolution fundamentally changes the availability profile of electrical energy due to the prevailing supply of renewable energy. The ESF-funded group of young scientists pursues the goal to balance regional differences in energy supply and demand through a temporal and spatial control of the energy consumption of data centers, which either happens via direct action or through dynamic pricing. This results in a reduction of fossil energy production and the involved CO2-emissions as well as an increase of the supply reliability without the necessity of an expansion of the energy grid. Within the interdisciplinary group of young scientists different competences are combined synergetically in order to achieve the goals of the project.
The main challenges of the project revolve around:
The main challenges of the project revolve around:
- handling of a complex overall system through hierarchization
- sufficiently detailed approximation of the nonlinear dynamics of the subsystems
- taking individual interests and goals of the subsystems into account
- integration of the energy market as a possible element for regulation
- optimal and hierarchical control of the subsystems and the overall system

BigApple is a large project that united five partners to systematically model, predict and control the apple value chain. The existing knowledge indicates correlations between certain factors such fruit growth, ripening, development of fruit diseases on all level of the value chain which include orchard, storage and sorting facilities. Since biological processes are complex, only fragmentary models of the said processes are available at the moment. It is however worthwhile to investigate dynamics of the value chain systematically in its generality. For this purpose, the Laboratory of Automatic Control and System Dynamics of the Technical University of Chemnitz utilizes its competences in modeling, regulation and optimization. The focus is particularly set onto methods that possess adaptation capabilities such as reinforcement learning. The apple value chain offers great potential for implementation and verification of such control strategies on the general level and also on the level of particular subprocesses such as harvesting and storage conditions. The key word of the project is “big data” which summarizes various techniques of manipulating large datasets, feature extraction for modeling purposes and consequently developing controls of the subject processes. BigApple uses different kinds of data, such as fruit growth, spectral images of apple trees, spectral assessment of ripening and damage to the fruit etc. All these data could reveal new correlations and help build new models of certain processes in the value chain.
The main focus lies in the following:
The major goals of the project are:
The main focus lies in the following:
- Identification of prediction and optimization potentials in value chain of apples
- control-oriented modeling of selected aspects of value chain via analysis of Big Data
- Developement and extension of adaptive and optimal control methods and their application in value chain
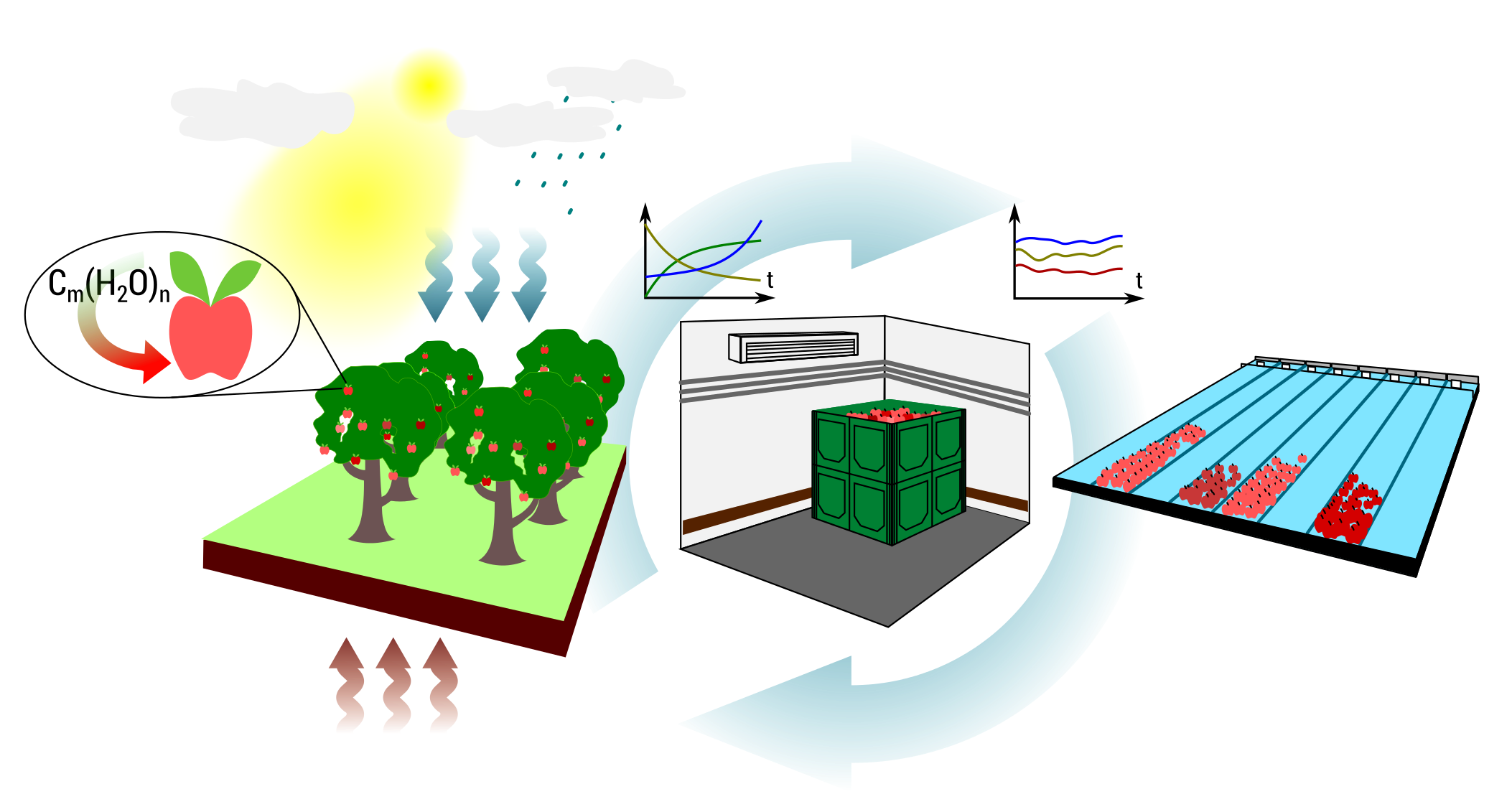
- Development of an apple production chain management system from the field to the consumer
- Energy savings to reduce the quantity and quality losses by means of a customized product and optimized harvesting and fruit storage
- Improved fruit quality production and quality maintenance for the benefit of consumers
- Strengthening the competitiveness of the German fruit growing and marketing organizations

With its primary supply role supermarkets are an essential part of the local grocery infrastructure. In the course of the energy revolution the high energy consumption of supermarkets also becomes of interest. Hence, supermarkets of the future will have to fulfill new requirements with respect to energy supply (electrical and thermal energy) in order to reduce energy costs (the main potential for savings). A long-term goal to be strived within this project is an energy efficient operation of supermarkets by means of an intelligent, partially automated and predictive energy management. This task is tackled by the optimal control of consumption and production, the dimensioning of cooling and heating systems, as well as ambient lighting and climate management.
Methodical challenges of the project are:
Methodical challenges of the project are:
- Time series analysis in order to identify energy efficiency potentials and to formulate efficiency criteria
- Combination of data-based and physically motivated modelling
- Model-based evaluation and diagnosis of the energy efficiency of supermarkets
- Automated diagnosis of the operations management in order to recognize abnormal behavior
- Combination of scheduling and underlying optimal control for the energy efficient operation of supermarkets
- Consideration of uncertainties (fluctuations, modelling inaccuracies, variation of operational parameters, etc.) via a stochastic model predictive control approach

A tractor, being the work horse of agriculture and the breadwinner of humanity, is perhaps one of the machines most vulnerable to depletion of fossil fuels. The problem of optimal tractor usage is a challenging control task. The major difficulty of it is a high influence of varying ground conditions on operation performance. Furthermore, energy efficiency and productivity of a tractor are two factors that not only depend on operation conditions in a highly non-linear manner, but they also play against each other. These facts raise a problem of a dynamical soil condition assessment and adaptive performance optimization methodology. The Laboratory presents a project “EiNES“ where the said problem is addressed based on the concept of a “sensor vehicle“: a means of real-time identification of ground conditions and digital communication for operator assistance and optimal machine control.
The project addresses the following goals:
The project addresses the following goals:
- development of smart process monitoring and data analysis methods for ground condition identification
- development of adaptive and learning optimal control algorithms for operator assistance and machine operation
- realization of an e-mobility-based sensor vehicle
- real-time ground condition mapping and assessment of biological fertility
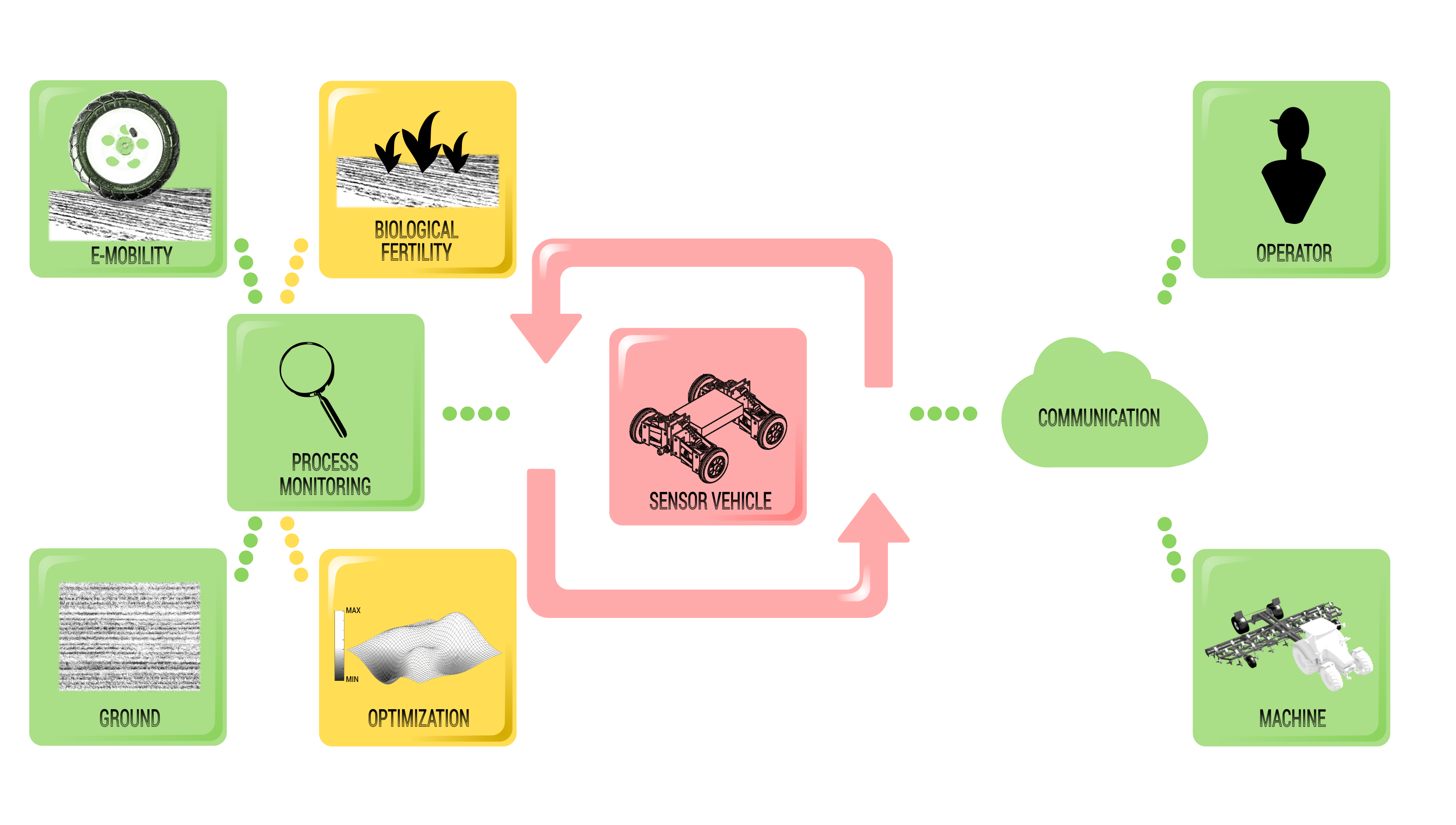

As a consequence of the energy transition from conventional to renewable energy sources, new technologies emerge. Hence, automatic control of electrical networks requires novel mathematical concepts and methods in order to describe and utilize flexibility potentials within and between network layers. Our goal is the consistent optimization and stabilization of the overall energy network system.
The specific focus of our group is a sophisticated model analysis and hierarchical control scheme using set-based methods and reduced-order models. This approach guarantees robustness and stability as well as consistency between the different levels of the hierarchical system.
The specific focus of our group is a sophisticated model analysis and hierarchical control scheme using set-based methods and reduced-order models. This approach guarantees robustness and stability as well as consistency between the different levels of the hierarchical system.

Food production is being challenged by various factors including climate change, growing population, decreasing groundwater levels, usable agricultural land, unpredictable weather, limited resources and the labor intensive tasks involved. The concept of indoor vertical farming (IVF) has proven to solve many issues involved with farming in open fields. However, the concept itself is continuously evolving to maximize the production by revisiting the greenhouse farming techniques and coupling the plant production with other agricultural systems (insects, mushrooms, fish, etc.).
The main focus of the project is to provide control and system theoretic methods to optimize the resource consumption and operation cost, and to provide robust and resilient operation of the agricultural system.
The major goals of this project are:
The main focus of the project is to provide control and system theoretic methods to optimize the resource consumption and operation cost, and to provide robust and resilient operation of the agricultural system.
The major goals of this project are:
- Control-oriented modelling of a small IVF system and coupled agricultural systems
- Modelling of hybrid energy systems to simulate energy and resource consumption
- Coupling of other biological systems for resource minimization by exploiting the symbiotic relationships
- Development of methods for optimal control and machine learning tailored for IVF

This projects addresses optimized nitrogen fertilization by multi-parametric data fusion and precise real-time application.
It aims at integrating the experience and expert knowledge of the farmer into a decision making and control algorithm by means of fuzzy logic.
This algorithm is capable of merging physical and information parameters of different nature into a unified format with the goal of diciding where and how much fetilizer should be applied on the field.
An important role plays the real-time biomass measurement since its distribution highly influences effects of fertilization.
Other crucial parameters include various map infromation such as yield maps.
Upon deciding on the place and amount of fertilizer, a to-be-developed application control systems serves precise and robust fulfillment of the fertilization objectives.
Therefore, methods of soft computing and artificial intelligence are used.
The overall setup thus amounts to a human-machine interface.
The project goals are:
The project goals are:
- to optimize nitrogen fertilisation of crop plants by using a data fusion of multiple parameters from offline maps and online sensors
- to minimize planning time for fertilisation by using a simple decision mask based on fuzzy logic
- to develop a model for the sensor-spreader system in order to improve the sitespecific application of fertilizer in terms of dose rate and distribution
- to increase nutrient uptake efficiency of crop plants and hence to minimize negative effects of greenhouse gas emissions

Being part of the HZwo initiative, the project Eco-CC is concerned with the development of a more economical and reliable concept of measuring and controlling low-temperature polymer electrolyte membrane fuel cells (PEMFC) for automotive applications.
Combining data from existing sensors with control-oriented dynamic models allows to increase the measurement accuracy without the need of developing new and expensive hardware.
Additionally, this software-based solution is capable of detecting faults and reconstructing missing data from other measurements, or might even allow to replace currently used sensors by virtual ones.
Specifically, the project addresses the following goals:
Specifically, the project addresses the following goals:
- development and validation of control-oriented dynamic models for specific components of a PEMFC-based power train
- analysis of the system's observability and indentification of optimization potentials wrt. required hardware components
- implementation of an identification and adaptation scheme for the online process
- analysis of sensor data fusion and the possibility of virtual senors
- implementation and test of a fault tolerant, optimal control scheme for PEMFC-based power trains
The focus of the research project is the implementation and investigation of a sensor system for the detection of nitrate and measurement of the nitrate concentration. In addition, a self-sufficient sensor network is to be developed with which it is both possible to supply the nitrate sensors with ambient energy, for example from sunlight or temperature gradients, as well as continuously acquire the sensor data and transmit it wirelessly to a central location for evaluation.
The developed sensor concept enables continuous monitoring of nitrate in the soil. Due to the self-sufficient energy supply, the sensor system operates maintenance-free and efficiently. By appropriate placement of the sensors and a suitable measuring point density, a field or operational assignment of nitrate entry is possible. This sensor thus serves both as a control tool for farmers to assess soil quality as a basis for fertilizer determination for the particular crop and nutrient input, and for the competent environmental authorities to assess the nitrate content in soils to protect the waters and to comply with the rules of fertilization after good professional practice, eg. compliance with distances to waters or fertilizer retention periods. From this requirement determination results in cost savings through the optimal fertilization with simultaneous soil conservation.
The system-theoretical aspects of this topic are:
More information can be found here.
- modelling and analysis of measurement dynamics in the presence model uncertainties
- optimization of sensors to increase the life span, reduce material degeneration, ...
- parameter and state estimation
- compensation of sensor faults
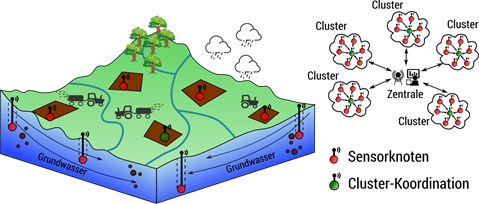
More information can be found here.
Besides population growth and climate change, urban growth is one of the largest challenges in the 21st century. Spatial and infrastructural borders between urban centers and rural areas disappear. Agricultural production needs to feed an ever-growing population. At the same time, current innovations in animal breeding and plant cultivation, as well as measures for increasing productivity are not able to compensate for the ever increasing scarcity of cultivation areas.
The vision of agricultural systems of the future is based on the idea that food will be produced in connected, mutually communicating and standardized production units, the so-called CUBES. Those CUBES are the basis for a closed food production system, which overcomes the weaknesses of earlier agricultural production systems by using ISO-standards, stackable units and a bio-cybernetic regulation approach. At the same time, the system integrates easily into the urban future. Due to its mobile nature, adaptability to a changing environment and an inherent scalability, the CUBES can be implemented in urban, rural and even desertified sites. Principles of closed cultivation methods will be integrated into a new process chain and the individual elements of the chain are intelligently connected and regulated. Thereby, synergies like the 'Triple Zero' concept can be used, enabling a production without additives and avoiding emissions and waste.
CUBES Circle represents, from control engineering perspective, a highly complex production system whose individual elements must be coordinated to realize closed energy and mass flows. In order to achieve this goal, modeling methods as well as model-based robust, adaptive and optimal control methods are considered. Mathematical formulation of dynamic models of the energy and mass flows of the CUBES-Circle will be used. These models will be parameterized and validated by means of parameter identification and machine learning/AI based on experimentally collected data from the production CUBES. Formulation of the quality criteria of the control techniques and the design of optimal control for energy and mass flow between the cubes will be based on the derived and analyzed models. It is important to synergistically compensate for the fluctuations that occur during operation (e.g. variations in cultivation, weather, variations in growth conditions) and to ensure stable and safe circulation. Based on the collected data from the production CUBES, the aim is to improve the models and control using Big Data analysis and learning methods. A combination of optimal, learning and adaptive control methods shall be considered to increase the efficiency as well as flexibility and adaptability of the CUBES Circle to new production sites and conditions.
More information can be found here.
The vision of agricultural systems of the future is based on the idea that food will be produced in connected, mutually communicating and standardized production units, the so-called CUBES. Those CUBES are the basis for a closed food production system, which overcomes the weaknesses of earlier agricultural production systems by using ISO-standards, stackable units and a bio-cybernetic regulation approach. At the same time, the system integrates easily into the urban future. Due to its mobile nature, adaptability to a changing environment and an inherent scalability, the CUBES can be implemented in urban, rural and even desertified sites. Principles of closed cultivation methods will be integrated into a new process chain and the individual elements of the chain are intelligently connected and regulated. Thereby, synergies like the 'Triple Zero' concept can be used, enabling a production without additives and avoiding emissions and waste.
CUBES Circle represents, from control engineering perspective, a highly complex production system whose individual elements must be coordinated to realize closed energy and mass flows. In order to achieve this goal, modeling methods as well as model-based robust, adaptive and optimal control methods are considered. Mathematical formulation of dynamic models of the energy and mass flows of the CUBES-Circle will be used. These models will be parameterized and validated by means of parameter identification and machine learning/AI based on experimentally collected data from the production CUBES. Formulation of the quality criteria of the control techniques and the design of optimal control for energy and mass flow between the cubes will be based on the derived and analyzed models. It is important to synergistically compensate for the fluctuations that occur during operation (e.g. variations in cultivation, weather, variations in growth conditions) and to ensure stable and safe circulation. Based on the collected data from the production CUBES, the aim is to improve the models and control using Big Data analysis and learning methods. A combination of optimal, learning and adaptive control methods shall be considered to increase the efficiency as well as flexibility and adaptability of the CUBES Circle to new production sites and conditions.
More information can be found here.