Daniel Brand
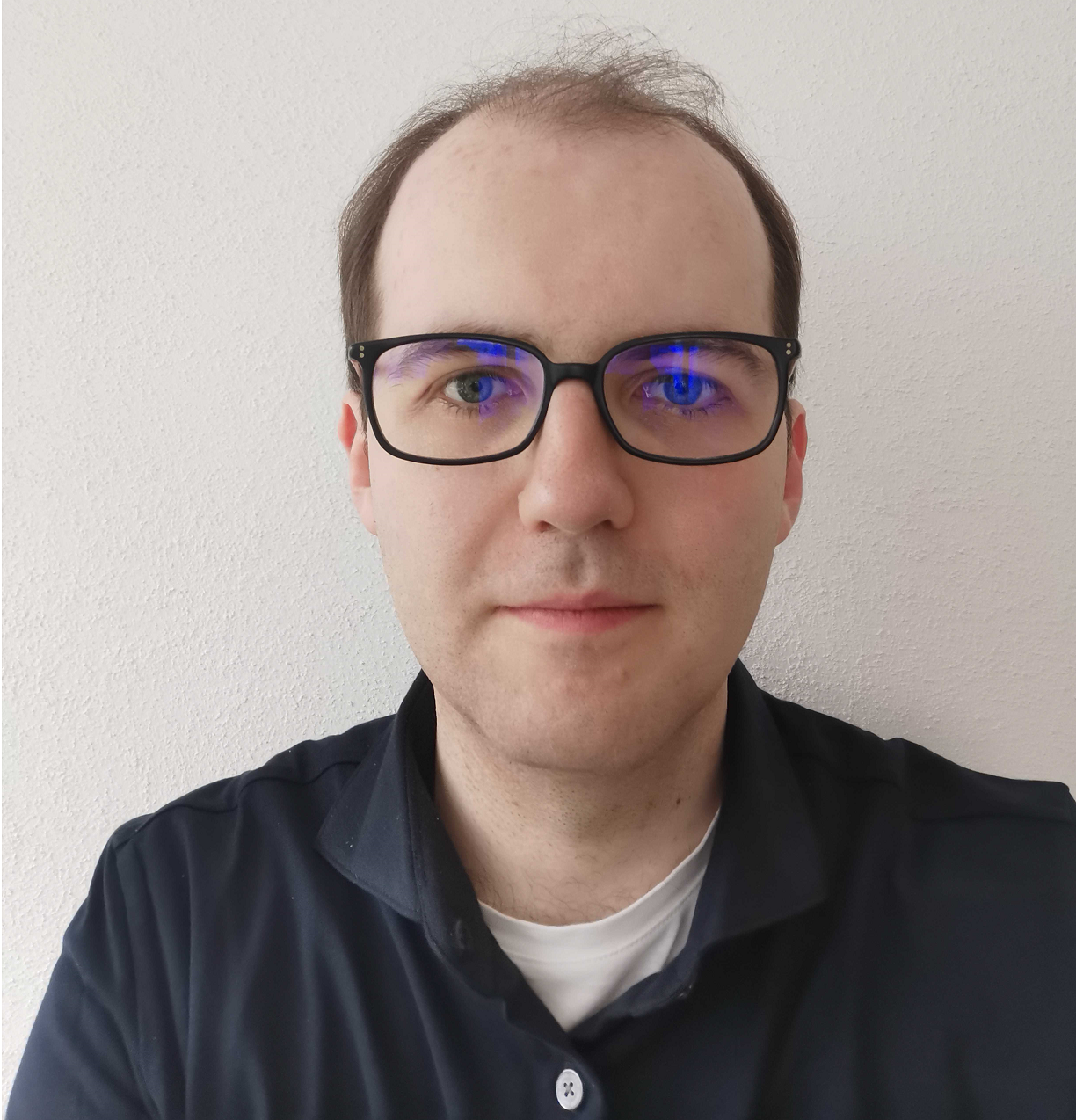
-
Telefon:+49 371 531-38957
-
E-Mail:
-
Sprechzeiten:Nach Vereinbarung. Bitte kontaktieren Sie mich per E-Mail.
Forschungsschwerpunkte
- Kognitive Modellierung
- Prädiktive Modellierung menschlichen Schlussfolgerns
- Syllogistisches Schließen
- Informationssysteme
Lebenslauf
Berufserfahrung
- seit 02/2022: Wissenschaftlicher Mitarbeiter; TU Chemnitz; Professur Prädiktive Verhaltensanalyse
- 02/2021 - 07/2021: Wissenschaftlicher Mitarbeiter; Syddansk Universitet (SDU); Abteilung für Design und Kommunikation
- 07/2020 - 02/2022: Wissenschaftlicher Mitarbeiter; Albert-Ludwigs-Universität Freiburg; Cognitive Computation Lab
- 05/2017 - 06/2020: Wissenschaftlicher Mitarbeiter; Albert-Ludwigs-Universität Freiburg; Center for Cognitive Science
Bildungsweg und Qualifikationen
- 2016: MSc. Computer Science, Albert-Ludwigs-Universität Freiburg
- 2012: BSc. Computer Science, Albert-Ludwigs-Universität Freiburg
Lehre
- SS 2022: Dozent; Seminar: Kognitive Ergonomie; TU Chemnitz
- SS 2021: Assistent; Seminar: Cognitive Modeling; Cognitive Computation Lab; Albert-Ludwigs-Universität Freiburg
- SS 2020: Assistent; Seminar: Cognitive Modeling; Cognitive Computation Lab; Albert-Ludwigs-Universität Freiburg
- WS 2019/20: Assistent; Seminar: Cognitive Reasoning: Methods, Algorithms, and Statistics to Discern Human from Artificially Generated Data; Cognitive Computation Lab; Albert-Ludwigs-Universität Freiburg
- SS 2019: Assistent; Seminar: Cross-Domain Modeling of Human Cognition; Cognitive Computation Lab; Albert-Ludwigs-Universität Freiburg
- SS 2014: Tutor; Cloud Computing; Department for Databases and Information Systems; Albert-Ludwigs-Universität Freiburg
- SS 2012: Tutor; Software Engineering; Department for Software Engineering; Albert-Ludwigs-Universität Freiburg
Projekte
Aktuelle Projekte
- Automatische Prozessmodellgenerierung für Kognitive Modellierung
Vorherige Projekte
Software
Publikationen
- Brand, D., & Ragni, M. (2023). Effect of Response Format on Syllogistic Reasoning. In M. Goldwater, F. K. Anggoro, B. K. Hayes, & D. C. Ong (Eds.), Proceedings of the 45th Annual Meeting of the Cognitive Science Society (pp. 2408–2414). [PDF] [GitHub]
- Todorovikj, S., Brand, D., & Ragni, M. (2023). Preferred Mental Models in Syllogistic Reasoning. In C. Sibert (Ed.), Proceedings of the 21th International Conference on Cognitive Modeling (pp. 252–258). University Park, PA: Applied Cognitive Science Lab, Penn State. [PDF]
- Brand, D., Riesterer, N., & Ragni, M. (2023). Uncovering Iconic Patterns of Syllogistic Reasoning: A Clustering Analysis. In C. Sibert (Ed.), Proceedings of the 21th International Conference on Cognitive Modeling (pp. 57–63). University Park, PA: Applied Cognitive Science Lab, Penn State. [PDF]
- Dames, H., Brand, D., & Ragni, M. (2022). Evidence for Multiple Mechanisms Underlying List-Method Directed Forgetting. In J. Culbertson, A. Perfors, H. Rabagliati, & V. Ramenzoni (Eds.), Proceedings of the 44th Annual Meeting of the Cognitive Science Society (pp. 519–525).
- Brand, D., Riesterer, N., & Ragni, M. (2022). Model-Based Explanation of Feedback Effects in Syllogistic Reasoning. Topics in Cognitive Science, 14(4), 828-844. doi: 10.1111/tops.12624. [GitHub]
- Mittenbühler, M., Brand, D., & Ragni, M. (2022). Do Models of Syllogistic Reasoning extend to Generalized Quantifiers?. In T. C. Stewart (Ed.), Proceedings of the 20th International Conference on Cognitive Modeling (pp. 189–195). Applied Cognitive Science Lab, Penn State: University Park, PA. [PDF] [GitHub]
- Todorovikj, S., Brand, D., & Ragni, M. (2022). Predicting Algorithmic Complexity for Individuals. In T. C. Stewart (Ed.), Proceedings of the 20th International Conference on Cognitive Modeling (pp. 240–246). Applied Cognitive Science Lab, Penn State: University Park, PA. [PDF]
- Kettner, F., Heinrich, E., Brand, D., & Ragni, M. (2022). Reverse-Engineering of Boolean Concepts: A Benchmark Analysis. In T. C. Stewart (Ed.), Proceedings of the 20th International Conference on Cognitive Modeling (pp. 164–169). Applied Cognitive Science Lab, Penn State: University Park, PA. [PDF]
- Brand, D., Dames, H., Puricelli, L., & Ragni, M. (2022). Rule-Based Categorization: Measuring the Cognitive Costs of Intentional Rule Updating. In J. Culbertson, A. Perfors, H. Rabagliati, & V. Ramenzoni (Eds.), Proceedings of the 44th Annual Meeting of the Cognitive Science Society (pp. 2810–2817). [PDF] [GitHub]
- Brand, D., Mittenbühler, M., & Ragni, M. (2022). Generalizing Syllogistic Reasoning: Extending Syllogisms to General Quantifiers. In J. Culbertson, A. Perfors, H. Rabagliati, & V. Ramenzoni (Eds.), Proceedings of the 44th Annual Meeting of the Cognitive Science Society (pp. 722–728). [PDF] [GitHub]
- Todorovikj, S., Kettner, F., Brand, D., Beggiato, M., & Ragni, M. (2022). Predicting Individual Discomfort in Autonomous Driving. In J. Culbertson, A. Perfors, H. Rabagliati, & V. Ramenzoni (Eds.), Proceedings of the 44th Annual Meeting of the Cognitive Science Society (pp. 3103–3109).
- von Stülpnagel, R., Findler, F., & Brand, D. (2022). Census-Based Variables Are Informative about Subjective Neighborhood Relations, but Only When Adjusted for Residents’ Neighborhood Conceptions. Sustainability, 14(8), 4434. doi: 10.3390/su14084434.
- Ragni, M., Brand, D., & Riesterer, N. (2021). The Predictive Power of Spatial Relational Reasoning Models: A New Evaluation Approach. Frontiers in Psychology, 12. doi: 10.3389/fpsyg.2021.626292. [GitHub]
- Brand, D., Riesterer, N., and Ragni, M. (2021). Unifying models for belief and syllogistic reasoning. In T. Fitch, H. Lamm, H. Leder, & K. Teßmar-Raible (Eds.), Proceedings of the 43th Annual Meeting of the Cognitive Science Society (pp. 2801–2807).[PDF] [GitHub]
- Brand, D., Riesterer, N., & Ragni, M. (2021). Model-Based Explanation of Feedback Effects in Syllogistic Reasoning. In T. C. Stewart (Ed.), Proceedings of the 19th International Conference on Cognitive Modeling (pp. 16–22). University Park, PA: Applied Cognitive Science Lab, Penn State. [GitHub]
- Mannhardt, J., Bucher, L., Brand, D., & Ragni, M. (2021). Predicting spatial belief reasoning: comparing cognitive and AI models. In T. C. Stewart (Ed.), Proceedings of the 19th International Conference on Cognitive Modeling (pp. 184–190). University Park, PA: Applied Cognitive Science Lab, Penn State. [PDF]
- Riesterer, N., Brand, D., & Ragni, M. (2020). Feedback Influences Syllogistic Strategy: An Analysis based on Joint Nonnegative Matrix Factorization. In T. C. Stewart (Ed.), Proceedings of the 18th International Conference on Cognitive Modeling (pp. 223–228). University Park, PA: Applied Cognitive Science Lab, Penn State. [PDF] [GitHub]
- Brand, D., Riesterer, N., & Ragni, M. (2020). Extending TransSet: An Individualized Model for Human Syllogistic Reasoning. In T. C. Stewart (Ed.), Proceedings of the 18th International Conference on Cognitive Modeling (pp. 17–22). University Park, PA: Applied Cognitive Science Lab, Penn State. [PDF] [GitHub]
- Riesterer, N., Brand, D., & Ragni, M. (2020). Do Models Capture Individuals? Evaluating Parameterized Models for Syllogistic Reasoning. In S. Denison, M. Mack, Y. Xu, & B. C. Armstrong (Eds.), Proceedings of the 42nd Annual Conference of the Cognitive Science Society (pp. 3377-3383). Cognitive Science Society. [PDF] [GitHub]
- Brand, D., Riesterer, N., Dames, H., & Ragni, M. (2020). Analyzing the Differences in Human Reasoning via Joint Nonnegative Matrix Factorization. In S. Denison, M. Mack, Y. Xu, & B. C. Armstrong (Eds.), Proceedings of the 42nd Annual Conference of the Cognitive Science Society (pp. 3254-3260). Cognitive Science Society. [PDF] [GitHub]
- Riesterer, N., Brand, D., & Ragni, M. (2020). Predictive Modeling of Individual Human Cognition: Upper Bounds and a New Perspective on Performance. Topics in Cognitive Science, 12(3), 960–974. doi: 10.1111/tops.12501. [GitHub]
- Riesterer, N., Brand, D., Dames, H., & Ragni, M. (2020). Modeling Human Syllogistic Reasoning: The Role of "No Valid Conclusion". Topics in Cognitive Science, 12(1), 446-459. doi: 10.1111/tops.12487. [GitHub]
- von Stülpnagel, R., Brand, D., & Seemann, A. K. (2019). Your neighbourhood is not a circle, and you are not its centre. Journal of Environmental Psychology, 66, 101349. doi: 10.1016/j.jenvp.2019.101349.
- Brand, D., Riesterer, N., & Ragni, M. (2019). On the Matter of Aggregate Models for Syllogistic Reasoning: A Transitive Set-Based Account for Predicting the Population. In Stewart T. (Ed.), Proceedings of the 17th International Conference on Cognitive Modeling (pp. 5–10). Waterloo, Canada: University of Waterloo. [PDF] [GitHub]
- Riesterer, N., Brand, D., & Ragni, M. (2019). Predictive Modeling of Individual Human Cognition: Upper Bounds and a New Perspective on Performance. In Stewart T. (Ed.), Proceedings of the 17th International Conference on Cognitive Modeling (pp. 178–183). Waterloo, Canada: University of Waterloo. [PDF] [GitHub]
- Riesterer, N., Brand, D., Dames, H., & Ragni, M. (2019). Modeling Human Syllogistic Reasoning: The Role of "No Valid Conclusion", In Goel, A., Seifert, C., & Freska, C. (Eds.), Proceedings of the 41st Annual Conference of the Cognitive Science Society (pp. 953–959). Montreal, QB: Cognitive Science Society. [PDF] [GitHub]
- Ragni, M., Dames, H., Brand, D., & Riesterer, N. (2019). When Does a Reasoner Respond: Nothing Follows?, In Goel, A., Seifert, C., & Freska, C. (Eds.), Proceedings of the 41st Annual Conference of the Cognitive Science Society (pp. 2640–2646). Montreal, QB: Cognitive Science Society.
- Riesterer N., Brand D., Ragni M. (2018) The Predictive Power of Heuristic Portfolios in Human Syllogistic Reasoning. In F. Trollmann & A.-Y. Turhan (Eds.), KI 2018: Advances in Artificial Intelligence. KI 2018. (Vol. 11117, pp. 415–421). Cham: Springer International Publishing. doi: 10.1007/978-3-030-00111-7_35. [Poster] [GitHub]
- Riesterer, N., Brand, D., & Ragni, M. (2018) A Machine Learning Approach for Syllogistic Reasoning. In C. Rothkopf et al. (Eds.), Proceedings of the 14th Biannual Conference of the German Society for Cognitive Science (p. 54). [Poster]
- Kottlors, J., Brand, D., & Ragni, M. (2012). Modeling behavior of attention-deficit-disorder patients in a N-back task. In N. Rußwinkel, U. Drewitz, & H. van Rijn (Eds.), Proceedings of 11th international conference on cognitive modeling (pp. 297–302). Berlin: Universitätsverlag der TU Berlin.
Vorträge und Posterpräsentationen
- "Effect of Response Format on Syllogistic Reasoning" @ CogSci 2023. Online, July 2023. [poster]
- "Uncovering iconic patterns of syllogistic reasoning: A clustering analysis" @ 21th International Conference on Cognitive Modeling. Online, July 2023. [slides]
- "Do models of syllogistic reasoning extend to generalized quantifiers?" @ 20th International Conference on Cognitive Modeling. Online, July 2022. [slides]
- "Model-based explanation of feedback effects in syllogistic reasoning" @ 19th International Conference on Cognitive Modeling. Online, July 2021. [talk]
- "Unifying models for belief and syllogistic reasoning" @ 43th Annual Meeting of the Cognitive Science Society. Online, July 2021. [slides] [poster]
- "How usable is Galaxy? A usability evaluation of Galaxy" @ 2019 Galaxy Community Conference (GCC2019). Freiburg, Germany, July 2019. [poster]
- "Extending TransSet: An Individualized Model for Human Syllogistic Reasoning" @ 18th International Conference on Cognitive Modeling. Online, July 2020. [short slides] [talk]